In April 2022, our research director Sabri Skhiri travelled to Zurich to attend the Privacy Enhancing Technologies Summit 2022, dedicated to PETs and their uses (enhance data security, facilitate compliance, and create value).
In this article, he gives you his opinion about PETs’s’ big trends, and a selection of his favourite talks.
Ready? Let’s go!
What are the PETs ?
Privacy Enhancing Technologies are the set of tools and processes that guarantee privacy within data processing or data exchange. The PET must be seen as enablers for creating value with data while complying with the law.
The main PETs are:
- FHE & encryption
- Differential privacy or anonymisation, and synthetic data generation
- VPN and security measures for “on the fly data” / “data in transit”
- GDPR & data governance: consent management, access management, retention period management, etc.
PART 1: The Big Trends
The conference audience was a mix between lawyers, security officers and techies. For the two first categories, the feeling was: “we are not tech, but we understand that blockchain, distributed ledger, AI and data are driving innovation in our verticals … how can we get a part of the cake ?”. For the techies, the main question was how to sell these technologies.
A second interesting element lies in the PETs’ pitches: speakers are not talking about cost-savings anymore but rather about data liability regarding contracts or regulations. But that’s not all. The main message is how data liability can enable value creation. They insisted on the “enabling” side of PETs to create value and open new perspectives. For instance, a lot of the storytelling was about the PET enabling partnerships between service or app providers who can finally create their own ecosystems and better create value. This is probably the message I have heard the most here: the next data science wave is about collaboration and data ecosystems. It involves three components: data science, data collaboration, and data privacy & confidentiality.
They were also a minority of talks presenting the PET as a risk management measure. The PETs can manage risks of non-compliance but also the risk of lack of trust from customers & reputation. However, this kind of storytelling was not as appealing as value creation for the audience.
I am personally worried about creating data sharing ecosystems. You typically need to gather companies who have a common objective to collaborate. A re-insurance company with insurers makes sense. But as soon as you have potential competition, most parties are concerned about sharing data. This is what is happening with the IoT world, where Samsung, Google, LoRa, sigfox have their own network and their own ecosystem. The risk is the fragmentation of the data landscape with siloed networks. To be continued…
PART 2: Take A Customer Perspective
The conference was the opportunity to see parties coming from:
- the academia trying to create PET,
- service providers who want to sell PET,
- and clients (Swiss RE, Swiss life, Here, Skyscanner, Banks, EU investment banks, ROCHE etc.).
The main conclusion is from the customers’ side. They are overwhelmed by the market: too many technologies are available, and customers do not understand the scope of the PETs and how to apply them. Moreover, the service and application providers expect clients to tell them about their challenges. But not many of them can really describe their challenges as they are lost between IT, technologies, regulation, business demand, digital transformation, AI & ML, etc.
The conclusion? Let’s stop talking about tech, let’s talk about customer ambitions, let’s discuss what their challenges are, and let’s even help them discover the challenges they need to tackle. We need customer empathy! We will discover that (1) the challenges are not the same for every customer, and (2) the solution will come from a kind of mix between data governance, privacy by design, and potentially PET (anonymisation, encryption, FL, synth data gen, etc.). This led a few speakers to propose the concept of a Privacy Enhancing Platform where data collection and distribution can be configured with a combination of PET technlogies.
PART 3: The Keynotes
Digital Markets & Ecosystem Level Collaboration: Outstanding Challenges to Data Monetization & Value Creation
Jags Rao works at the Swiss Re Group, one of the world’s leading providers in the reinsurance and insurance business. They have existed since 1896 and collected a vast amount of data that represents decades of knowledge acquired about risk and risk management. They were exploring how to use this knowledge to create value today? How to formalize this knowledge?
Data ecosystem
According to Jags Rao, the three main barriers to the establishment of an ecosystem for value creation using data are
- Accessing to data
- Accessing to a critical mass of customers (more than enough to go forward than each peculiarity)
- Creating a Minimum Viable Ecosystem (MVE) Partners: building an ecosystem enabling you to reduce the cost and risk of innovation by ten. It is no longer up to you alone to innovate, and the risk of innovation is smoothed out over all the partners. Moreover, if you are a large company, you can let smaller, more agile structures accelerate the innovation life cycle. All in all, a win-win situation!
Once you get this, it naturally leads to business innovation opportunities for re insurance:
- Minimum viable product
- Risk as a service API: digital insurance covers and risk service
- Platform for fee-based business: orchestrating the re-insurance business
Fundamental challenges:
- Data liability: violation of contractual & regulatory liabilities
- Data integrity & security: data theft, data breach, malicious access
- Value exctrability : liability or risk incurred during value extraction
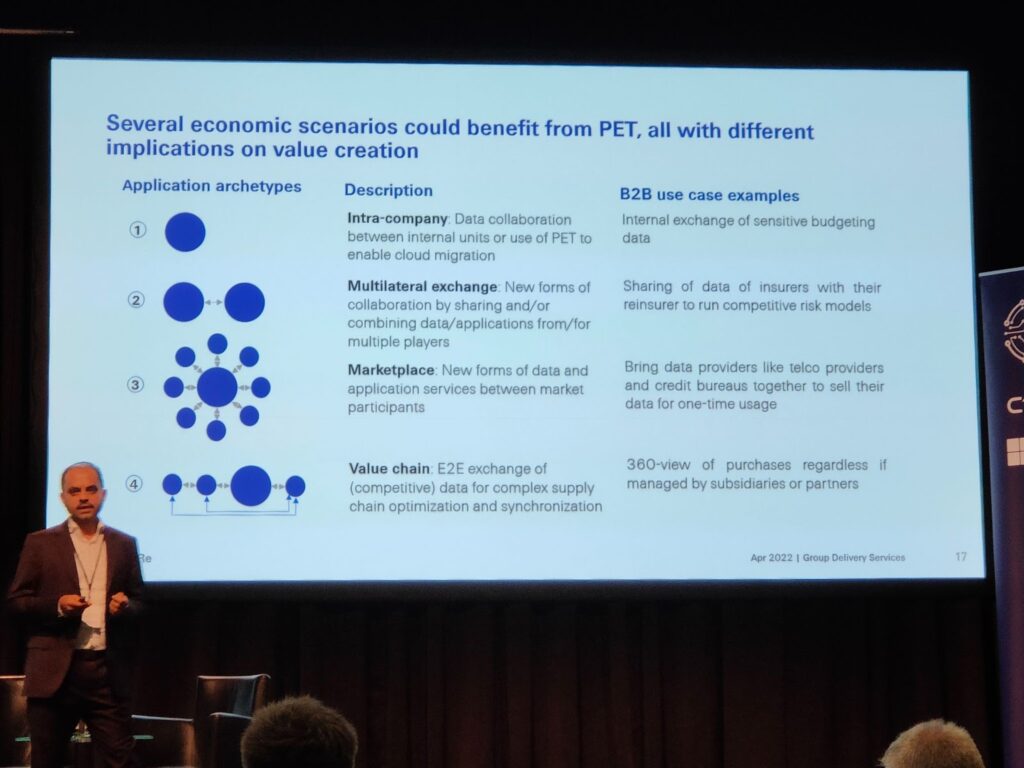
But along these 3 challenges, we still need to provide two mandatory features: explainability and metadata management.
Jags Rao concludes with several scenarios that could benefit from PET, all with different implications on value creation.
- Intra company: when you are dealing with private and sensitive data within your company and when you need to check the data usages.
- Multilateral exchange: when you need to exchange data with partners or daughter companies.
- Marketplace: when you want to set up data marketplace to foster data exploitation in your value chains
PART 4: Favourite Talks
Talk 1: Top 3 Lessons Learned When Helping Global Organizations Mitigate the Privacy-Utility Trade-Off
Although it was a sponsored talk, I liked the three lessons learned shared by the speaker (Jordan Brandt, Cofounder and CEO at Inpher).
1. Define the problem and the success metrics before choosing a privacy tech
For example, in banks, BNY Mellon achieved more than 20% improvements in fraud detection rate with ML models trained on internal data. But, when BNY used counterparties data, they got an additional 5 to 10% improvement through collaborative PPML (privacy-preserving ML). The speaker concluded that counterparty data enriched the accuracy of the model.
Defining the problem is mainly about asking the right questions:
- How many data sources?
- What data privacy sovereignty laws apply?
- What type of analytical operations/models are required?
- How is data distributed and joined?
- What is the dimensionality?
- What degree of model accuracy is required to achieve the ROI objectives?
2. Integrate and orchestrate PET in the end to end data pipeline, control and audit. Do not leave your PET alone.
The recommendation here is that the PET should be part of a more general program that includes data governance, data architecture and technical implementation. In addition, a mix of PET will be required in some contexts.
For example, if you want to get companies to work together on data, you have to share it in the right way and therefore use either consents or contracts or generate synthetic data or use encryption.
3. Collaborate with partners to build and refine secure data ecosystems
Ex: banks, asset managers, healthcare, and AdTech organisations are leveraging PET to build their own ecosystems.
Jordan Brandt presented the concept of secret computing, where encrypted data can be used in analytics and ML models. He argues that the accuracy should be better with more data: from intra to inter-department and finally to the ecosystem of data partners. He also claims that the best ratio between privacy and precision is the MPC (multi-party computing) and FHE (fully homomorphic encryption) (just after federated learning use case).
Talk 2 : Bridging the “Data Gap” Quickly & Efficiently
Bilal Husein’s (Data Analyst at Roche) tag line was: do your tax declaration yourself!
I really liked this slogan! People tend to pay someone to do their tax declaration…
Why? Lack of literacy, lack of knowledge and education. It’s actually the same thing in data science with business analysts!
Bilal Husein argued that data and analytics literacy is mandatory for the business sector: exactly as for a tax declaration or even a floor plan architecture drawing. Everyone has the literacy to read such a plan. The same should be true for reading a correlation matrix or the ability to join data “à la” tableau. Consider the example of a “what if analysis”. If we lower the bid price by X, what is the probability of winning a tender? I should be able to see the simulation for X from 0 to Xmax, which gives 100% probability of winning the tender. This literacy will also help you to smell the right opportunities. But this also means that, as an analyst, you should be a Swiss knife of ML: NLP, graph, Statistics, synthetic data, etc.
The speaker also came back to the tradeoff between utility and privacy. His answer was “it should be adaptive“, depending on what you want to have. Do you want internal, cross-company, open data? What kind of data? Once you have the answers to these questions, you should be able to define what is an acceptable level of tradeoff.
Finally, Bilal Husein presented his vision of clean rooms: first, share the data schema, and then you can code what you are looking for (average, STD variation, …), and apply the code without seeing the data, and finally the result is coded. In my opinion, this is fine as soon as you have a legal ground for acquiring the data, but what puzzle me here is that it looks like a data processing. Not seeing the data is not the final goal of the GDPR, but rather to limit the data processing.
Talk 3 : Accessing Data at Scale to Power Precision Medicine Through Privacy Preserving Machine Learning Techniques
I like this talk because it discusses how we can do efficient federated learning. The speaker, Mathieu Andreux, works at the Owkin Federated Learning Research Group. They have shown that applying in a naive manner the method leads to poor results.
The main use case for federated learning is about hospital data integration: due to data sensitivity, health data is hard to share and often lies in silos, preventing AI models from being trained on large datasets. The idea is to train a model locally in the hospital that generated the data and to share local models at the aggregation server level.
Mathieu Andreux showed a couple of use cases, such as the breast cancer health chain (can we predict the rate of pathological complete response to neoadjuvant chemotherapy ?). Everything, like image processing and predictive models, are done at the hospital level.
They also work on the MELLODDY project: a network of 10 pharmaceutical companies collaborating to train machine learning models for drug discovery based on private and highly sensitive screening datasets (including 10 million annotated molecules, 1 billion assay biological activity labels). Owkin’s platform allows securing the model aggregation without compromising the security nor disclosing anything about each participant’s data.
Challenges and research direction
- Distributed ML: aggregation method
- Statistical heterogeneity
- Privacy
Finally, the speaker showed that the performances are quite poor when naively applying federated learning. Batch normalization can significantly increase the performance. Privacy can be tackled with secure aggregation or differential privacy (secure aggregation is a kind of data compression that optimizes communication). Of course, here we speak about model parameter encoding.
Talk 4: Real Applications of Synthetic Data in Modern Organizations
I liked this talk because the story telling was quite close to what want to do in our research track about data anonymisation.
Everything starts will real data. Data is at the core of every innovation, but there are a couple of challenges:
- Talent and skills shortage for collecting and using data
- Uncertain RoI on upfront investment
- Data quality, availability, especially missing data
- Regulation on data protection see the following map (source DLA piper 2022)
Where synthetic data can help?
We can generate artificial data by learning the characteristics of the source data. We can estimate quite accurately the distribution and generating very realistic data.
Different usages:
- Insight: ML models, complete missing data, and allows using data without privacy concerns.
- Innovation: test/ dev, exploration
- Business: sharing, selling data.
Synthetic data as a PET:
- Sensitive data classification
- Extensive utility testing
- Re identification risk evaluation
- Complementary privacy mechanism
Coffee discussion:
During the coffee break, I had the opportunity to discuss with the CEO and co founder of Statice, a tool that generates synthetic (claimed privacy preserving) data. Only on tabular data.
You know me, I deep dived into a few tech details:
- No graph synth generation, not at all evolving graph nor for evolving tabular data
- Nothing for minority representation
- SDK allows adapting the level of noise, but they stop there. There is nothing to drive something like a privacy score.
- No factual results on privacy attacks.
- The fact that minorities are either delated or amplified in the synthetic process generation is not something they can easily deal with
Talk 5: Using Confidential Computing in Finance, Healthcare, Government & Other Industries
This is a very interesting new concept in cloud computing when you need to highly secure your data and your environment. I am pretty sure it will become more and more critical for our customers.
As the speaker (Manuel Costa, Partner Research Manager) explains, the starting point for Microsoft is the Cloud. You have different environments, different physical locations and physical accesses. It is a fairly complex environment. Microsoft claims that everybody is moving to the Cloud and, as a result, privacy has become highly important.
Their customers want full control over data:
- Privacy
- Untrusted collaboration
- Minimize trust: they would like to minimize the number of components they trust in the cloud, which leads to the concept of zero trust env
- Regulation and compliance
The existing encryption covers mainly data at rest and data in transit. But as soon as you decrypt and use data, you are under threat. This is why confidential computing goes one step further by protecting data in use. This is going quite far by even protecting data in the guest OS, Host OS kernel, hypervisor, and even letting sharing data across multi parties.
The basic concept is the Trusted Execution Environment
It allows isolating the code and the data of a given confidential workload from any code running in a system with encrypted memory. That means that even if your machine is hacked, everything is encrypted.
They propose the concept of the attestation service to share your environment with multiple parties. (See picture) Going further, even the key management system can be implemented within a trusted execution env.
Microsoft announced the availability of Confidential VMs, Container, and now open Enclave SDK to create your own custom secure env. The also launched new Azure confidential computing services:
- Azure confidential ledger
- Azure key vault management HSQM
- Azure SQL Always Encrypted (AE) with secure enclaves
Conclusion
It was an excellent opportunity to visit a nice city and make the link with privacy by design: very interesting talks and panels! But there are still many interesting questions: how to make factual the trade-off between privacy and utility? How to use PET with deep neutral nets & non-linear processing? What are the main legal/PET requirements when sharing data outside your company or across borders when different sovereignty laws can apply? Insightful workshop!