Before being a trendy expression, “Trustful AI” represents a primary need in machine learning: how can I trust a model and its decisions? Without an answer to such a question, the adoption of machine learning models is complicated.
To address this problem, we started a new collaboration with Aix-Marseille University, Montreal University, Nantes University, and St-Etienne on a four-year project called TAUDoS. Our research engineer Nicolas explains:
“Amongst other things, trust can be brought by interpretability: “What made my model make a certain decision?” Explaining decisions is especially hard for models trained on sequential data (time series, natural language, …), and there is still a lot of work to do in order to have a general well-behaved solution. Finding such an approach is the aim of the Taudos project.”
Last week, the whole consortium met in Marseille, to discuss findings, future directions, and challenge ideas. Multiple talks for both theoretical and industrial settings have been presented. The main research direction is to find links between automatas (well-known interpretable models created a long time ago) and modern, less interpretable approaches.”
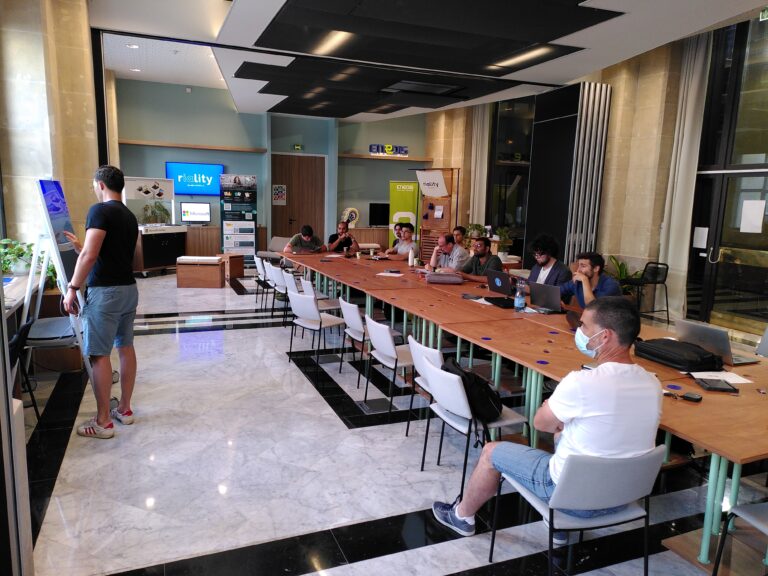
The TAUDoS project also supports the ambition of our two-year research program, BISHOP, which aims at addressing the challenges of responsible artificial intelligence, and ensure data confidentiality and trust in AI models.