This November, our research director and senior researcher Sabri and Gianmarco took part in the kick-off of a TETRA project led by KU Leuven that aims to develop a methodology for combining data from different sources to solve complex configuration problems in a decentralised way.
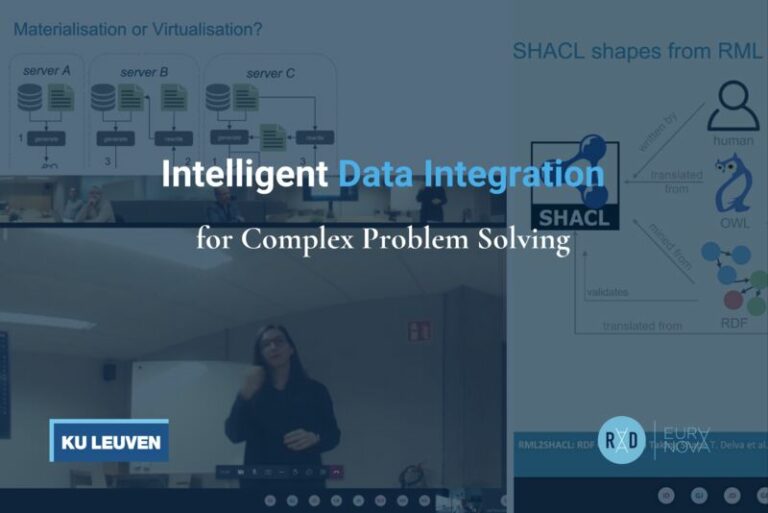
Sometimes, knowledge needs to remain decentralised. A typical example is a health care system that requires making a decision for a patient. The decision process might be complex and needs information from multiple sides that cannot be centralised.
Yet, in front of complex problems, many interesting applications require data from different sources.
A few months ago, the KU Leuven and Anastasia Dimou asked Euranova to bring its expertise to their new project: develop a methodology for
- capturing knowledge from different sources
- without physically merging the data
- and considering GDPR constraints.
If achieved, it will provide sophisticated decision support in a variety of domains, such as medicine, finance, telecommunications or marketing.
In the upcoming months, we will work to determine the future directions, including the technologies to investigate, the case studies to develop, and the training to organise
Very excited to embark on this new adventure!