In June 2022, our research director Sabri Skhiri and the head of our data science department Madalina Ciortan travelled to Copenhagen to attend DEBS 2022, the leading conference focusing on distributed and event-based systems.
Madalina was invited to the Industrial Workshop to talk about the industrial challenges of graph neural networks.
Large network data evolving over time have become ubiquitous across most industries, ranging from automotive and pharma to e-commerce and banking. Despite recent efforts, using temporal graph neural networks on continuously changing data in an effective and scalable way has been a challenge.
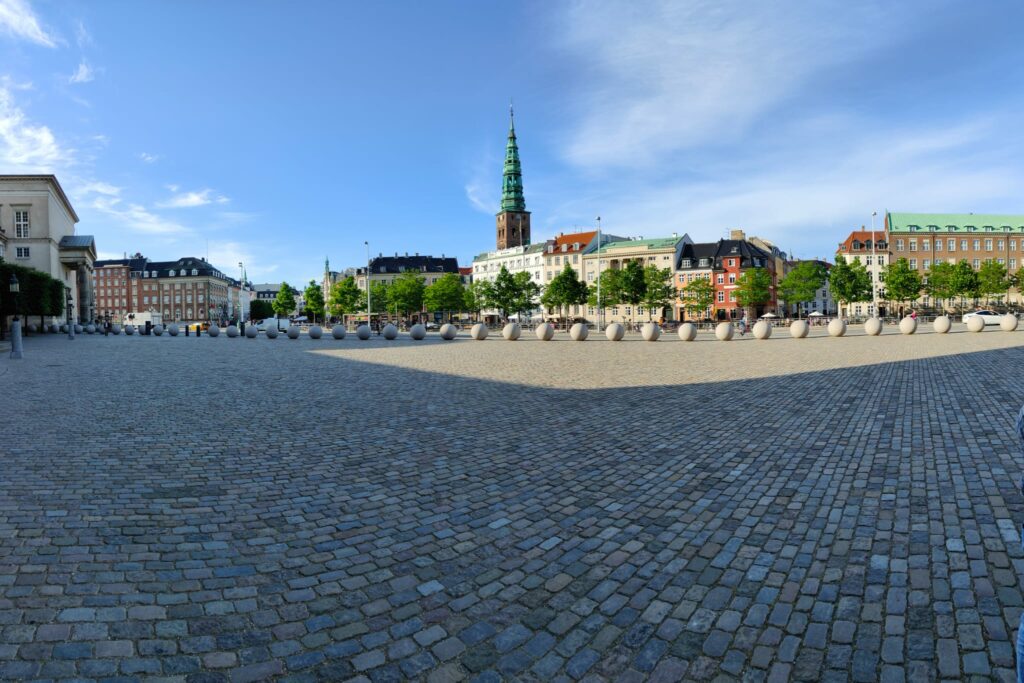
She presented one of our new research tracks called Dilithium, which aims at addressing the challenges of time-dependent graphs. We provide an overview of relevant continual learning methods directly applicable to real-world use cases. As explainability has become the central ingredient in trustworthy AI, we also introduce the landscape of state-of-the-art methods designed for explaining node, link or graph level predictions.
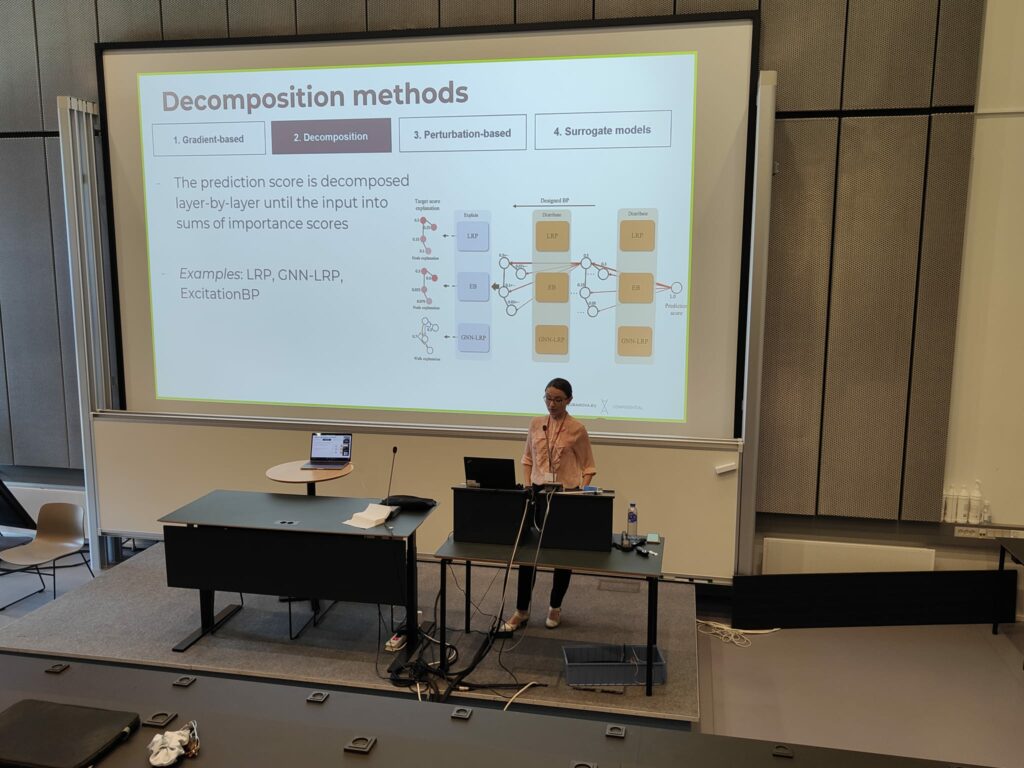
Wished you were there?
If you missed her talk, you can find the slides of her presentation here.
Big thanks to DEBS committee for the great organisation and for bringing all this the stream community together!
The event was packed with a lot of good discussions and insightful talks. We are very glad to have been part of it! As Sabri said: “A great week at the DEBS conference talking high performance and distributed systems and a city tour in Copenhagen…what else?”